Forward-Looking Panel on the Future of CSE

By Karthika Swamy Cohen
Where is computational science and engineering (CSE) headed? What new “grand challenges” will stimulate progress? How will the field benefit from machine learning and scientific computing? How will it drive new application areas like computational biology and medicine, computational geoscience, and materials science? What opportunities and challenges may we encounter in extending CSE to new subjects such as social network analysis, cybersecurity and the social sciences?
Experts in the community hailing from diverse application areas addressed these important questions at the forward-looking panel during the SIAM Conference on Computational Science and Engineering, happening in Atlanta, GA this week.
As moderator George Karniadakis put it, “We have 75 minutes here to forecast the future of CS&E.”
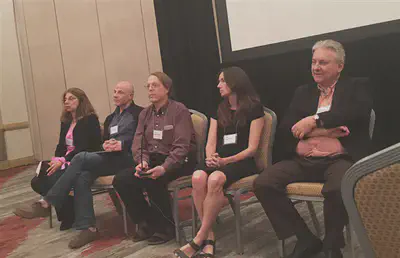
Horst Simon, deputy director of Lawrence Berkeley National Laboratory (LBNL), emphasized the field’s universality by discussing its extensive utilization. “I encounter CSE problems almost on a daily basis,” he said.
Simon went on to describe the federal Brain Research through Advancing Innovative Neurotechnologies (BRAIN) Initiative at LBNL. “We put electrodes in patients’ brains and see if we can actually see how someone speaks words,” he said.
With a proposed budget of $4.5 billion over 12 years, the BRAIN Initiative aims to facilitate discoveries and breakthroughs in our understanding of how the brain works and develop tools and technologies to prevent, diagnose, and treat brain and neurological diseases.
At the other end of the application spectrum, CSE helps address these challenges. “How can you squeeze water out of paper?” Simon asked. “From the brain to the practical, CSE is great.”
He also talked about machine learning and artificial intelligence (AI), referencing the AI accomplishment about which nearly everyone has heard: the victory of DeepMind’s AlphaGo over human Go champion Lee Sedol.
“Machine learning and AI are super hyped today, but it’s just another tool,” Simon said. Future growth will depend on performance improvements in hardware platforms, algorithms, and parallel implementations. He also made a plea to computational mathematicians. “Please embrace quantum computing,” he implored. “Don’t let physicists take over quantum computing.”
Simon then mentioned two Obama administration projects, which are unlikely to progress due to budget cuts in the Trump administration: the National Strategic Computing Initiative, which has no attached funding, and the Exascale Computing Project. He insisted that the CSE community and SIAM must embrace the latter, whose key objective is attaining a factor of 100 improvement for about 25 previously-selected applications. “This is true application work - it is real CSE,” Simon said.
Omar Ghattas, a computational geoscientist from the University of Texas at Austin, spoke about the use of unprecedented amounts of data and computational models in the field of climate science. “How do we capitalize on this relentless growth of computing power?” he asked. Ghattas added that with rapidly expanding volumes of observational data, models don’t get as much love as they used to. “There is this popular view that we don’t need mathematical models, and that big data and machine learning will obviate all of that,” he said. “I don’t think it needs to be said, but big data alone is not going to allow us to understand our planet and predict its behavior.”
Science and technology advance through the interplay of data and models, each propelling the other. “Observations push theory, theory pushes observation, and we move forward,” Ghattas said.
The modern approach of integrating data and models has been working well in climate science, he continued. This is why weather predictions have steadily improved over the past decade. Improved models improve data.
Ghattas then explained how to integrate all of this current observational data into a model. “The answer is it’s an inverse problem,” he said.
We need methods to extract knowledge from data while respecting known physical laws and constraints. The challenges to this strategy are manifold. Model parameters often represent infinitedimensional fields. Many inverse problems are ill-posed. Data tend to be noisy and sparse. Models are often inadequate and uncertainty becomes a fundamental feature of these problems.
Enter the Bayesian inference framework for inverse problems. “Bayesian inference provides a coherent and systematic framework for addressing these challenges,” Ghattas said. “The holy grail I see [in this field] is that we will be able to take all this observational data with uncertainties and put it all together in a Bayesian framework.”
Fariba Fahroo, program manager at the Defense Advanced Research Projects Agency (DARPA), spoke about the importance of CSE to DARPA projects. “I can see CS&E being relevant to everything they’re trying to do,” Fahroo said. “What’s so unique about DARPA for me is that its mission is supposed to be creating surprises and preventing surprises in the areas of science and technology and national security.”
She noted synthetic biology, biomedical devices, and neuroscience as specific fields that have much use for CSE tools.
Fahroo echoed Simon’s thoughts on emerging areas like big data and machine learning. “The hype that they have received has puzzled a lot of people who have dealt with big data and machine learning,” she said. “They are very surprised that the field has become so hot.”
However, she insisted that computational scientists should ignore the hype while simultaneously owning these areas. “This is something we should embrace, and just make the community aware that we have the skills for it,” Fahroo said.
She mentioned that data-driven modeling as another very interesting area of growth for the DARPA community. Individuals with a background in computer science are embracing it, thus essentially taking traditional modelers, such as physicists and chemists, out of the modeling business.
Fahroo then went on to explain a few DARPA programs of relevance to CSE. Enabling Quantification of Uncertainty in Physical Systems (EQUiPS) provides a rigorous mathematical framework for quantifying, advancing, and managing multiple sources of uncertainty in the modeling and design of complex physical and engineering systems of interest to the Department of Defense.
The end-to-end uncertainty quantification process is one aspect in which to engage the CSE community, Fahroo said.
Models, Dynamics and Learning (MoDyL) is another pertinent DARPA program that aims to build rigorous data-driven models for non-equilibrium dynamics, which can address challenges posed by complex, nonlinear, multiscale dynamical systems. These systems often evolve to a critical state due to irreversible and unexpected events, severely limiting mathematical model development and implementation for accurate prediction, formation, and pattern evolution.
Karen Willcox, professor of aeronautics and astronautics at the Massachusetts Institute of Technology (MIT), spoke about how CSE helps build the next generation of aerospace vehicles. Aerospace vehicles have increasing levels of autonomy. New technologies, data, and computational abilities inspire new ways of vehicle design for efficiency, cost, reliability, adaptability, and performance. Aerial vehicles are becoming interconnected and self-aware. “In a sense, vehicles will know about structural health as a human does,” Willcox said. “They’ll know how they feel in any given day and what that might mean to the way they fly.”
Virtual clones have greatly advanced aerospace, and virtual models informed by physics-based models and system-specific lifecycle data are blurring the boundaries between design, manufacturing, and innovation. This fluidity allows researchers to model at various scales and assures new approaches for decision-making.
“Even virtual models of ourselves change the way we think about healthcare and what we eat on a given day,” Willcox said.
The next generation of engineering systems will need predictive models, predictive data surveying, scalable uncertainty quantification, scalable methods that exploit data and models, and robust and sustainable software tools.
“If you take away the adjectives on this above list, we have all of these today,” Willcox said. “But we need to keep thinking about them.”
In short, unprecedented sensing capabilities and onboard computational power are changing the way researchers think about aerospace.
David Bader, professor and chair in the School of Computational Science and Engineering at the Georgia Institute of Technology, presented an overview of the school’s history and mission.
With a focus on solving real-world challenges, the School of Computational Science and Engineering emphasizes advanced computational techniques. It promotes innovation in computational methods and data analysis practices that solve diverse problems in areas such as cancer and disease diagnosis and treatment, sustainability, transportation, social networks, national security, and defense. A main objective is the development of novel techniques for large-scale computation and massive data sets. The school often partners with leading industrial organizations and national labs, essential to progress in all research areas.
“We put together the school that we all thought we wanted when we were kids,” Bader said.
He talked about addressing globally-significant grand challenges and noted that emerging trends lead toward new architectural features.
Possible applications of CSE range from healthcare, massive social networks, and intelligence to systems biology, the electrical power grid, and modeling and simulation, Bader said.
The panel then took questions from the audience, addressing queries about CSE education, workforce training, and “Grand Challenge” issues.
https://sinews.siam.org/Details-Page/forward-looking-panel-on-the-future-of-cse